Concept of GD.findi
- Home
- /
- Technologies
- /
- Concept of GD.findi
The Turning Point of Manufacturing
In accordance with the swift manner with which the borderless economy has spread, the way in which manufacturing is performed has been greatly changed. From here on, development of an infrastructure and management strategy to support global manufacturing will be of utmost importance to the future of manufacturing
As manufacturing requests of a high-variety, low-volume nature are becoming more frequent along with the expectation of shorter lead times, more stringent inventory and cost control procedures are required. However, cost reduction and organization streamlining result in younger employees being unable to learn the traditional wisdom and know-how of the manufacturing industry. Should this continue, the existence of manufacturing professionals will cease. Manufacturing organizations should be strengthened to foster competitiveness, innovation, and pride within the industry.
On the other hand, in the world of manufacturing and process automation technological phenomena such as Industry 4.0 and IoT (Internet of Things) are beginning to see use in production environments. These technologies leverage the power of ICT to its fullest, and possess a disruptive power capable of changing even the foundations of the manufacturing industry.
In light of the current situation and movement towards ICT, is exploring challenges in production technology and manufacturing departments, and are developing a web service as the realization of this approach. In this volume, the concept behind and background information underlying GD.findi are described to help elucidate an approach to future manufacturing procedures in the reader.
"The Professionals are Gone!!"
The structure of production departments in the manufacturing industry remained unchanged in the face of widespread globalization. However, it is not only a restructuring that is required at this stage. The introduction of Western-style management and business practices such as cost reduction, cutting lead time, and saturating market verticals resulted in a need for developing products of a wide variety. Such is the underlying cause of the change from mass-production to high-variety, low-volume operations. With the increased pressure and results-oriented nature of operating, the reliance on outsourcing increased and promoted an atmosphere in which specialization was valued. But the narrow range of expertise afforded by these technical personnel combined with the aging of the engineers will lead in a gradual loss of knowledge within the company. This is responsible for both the decrease in quantity as well as quality of technical staff.
Furthermore, the continuation of the current state of affairs will result in a situation wherein technology is seizing on a massive scale the foundation of production itself. The transition of the manufacturing industry into such a state will result in the “manufacturing professional” becoming all but extinct as a career. In order to maintain a workforce of manufacturing professionals, it is crucial that companies sustain and grow an internal culture of the essence of manufacturing, lest it fade away and be forgotten.
A professional is an individual who fosters intelligent engineering activities systematically, and draws upon a wealth of experience in these actions. They are not merely a craftsman with skill. However, in the current state of the manufacturing industry, it is not a highly specialized workers that are responsible for solving recent problems, but rather technology that has performed the intellectual problem-solving activities. As a result, this type of professional has become an endangered species in the marketplace. Up until the collapse of the Lehman Brothers, there was an extreme focus on mass-production and there was no leeway permitted for innovations within the field. These scarce experts in the field of manufacturing will become increasingly important as time progresses to leverage their wisdom for the sake of progress.
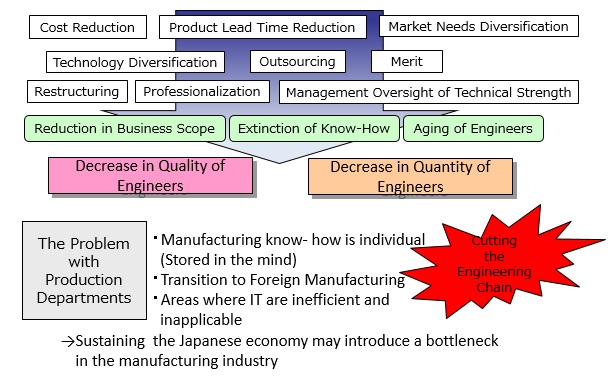
Manufacturing Strategy from a Production Systems Standpoint
>The Matter of Improvement Strategies
Until now, the baseline model for manufacturing capabilities was by “improvement”, especially in Japan. For a long time, the traditional idea of improving quality by producing large volumes of a product was followed. However, if the current manufacturing industry is diversified by entering a stage in which high-variety, low-volume production schedules come into favor it is of utmost importance that existing assemblies specializing in high-volume, low-variety production adapt to new products without a drop in product quality.
When examining the process of “improvement”, it is important to realize that “improvement” is an important approach. On the other hand, these “feedback” phenomena pose important questions which must be carefully considered. Feedback is a problem which simultaneously considers the output of the system, thereby eliminating the need for re-tooling. Due to the fact that there are no present alternative solutions, “improvement” is still an indispensable technique. However, because “improvement” is more than a simple rework, it goes without saying that situations where it is not required are desirable. Taking into consideration today’s marketplace, where demand for lower-fidelity prototypes is increasing before reaching the mass-production stage, it is essential that a solution is developed to alleviate this problem.
Furthermore, when a high-variety, low-volume production lot is requested, certain aspects of production line configuration such as inventory and inter-procedural operations become much more difficult to improve.
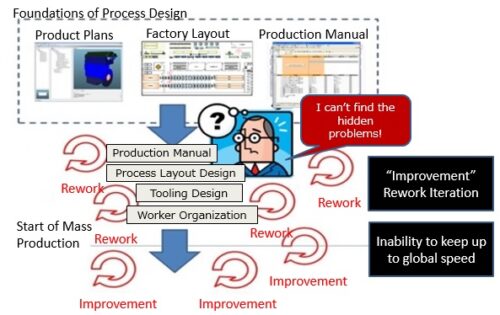
>Feed-forward Production Systems
Although it is important to continue to consider the role of improvement in the manufacturing process during the migration period from mass-production to high-variety, low-volume production, the application of a “feed-forward” mindset, whereby the best of measures are implemented in advance so as to prevent problems, is becoming increasingly important.
Continuing to confront such problems by iteration will become increasingly difficult, as the highly differing components required in high-variety, low-volume manufacturing must be taken into account. As such a technique requires considering only the factors relevant to a specific task, it is difficult to apply in a rapidly-changing environment such as one afforded by high-variety, low-volume production. As feed-forward makes a point to establish solutions to such problem well in advance, it is better-suited for generating appropriate guidance in designing manufacturing operations in such environments.
Furthermore, the simultaneous application of feed-forward and feedback methodologies as a “twin strategy” permits leverage of knowledge from both the past and future and application of said knowledge in the present. Management guidelines and production tactics feed-forward, and when combined with the accumulated know-how in production departments can be shared with the business management departments. This creates an information loop whereby communication and flexibility are increased.
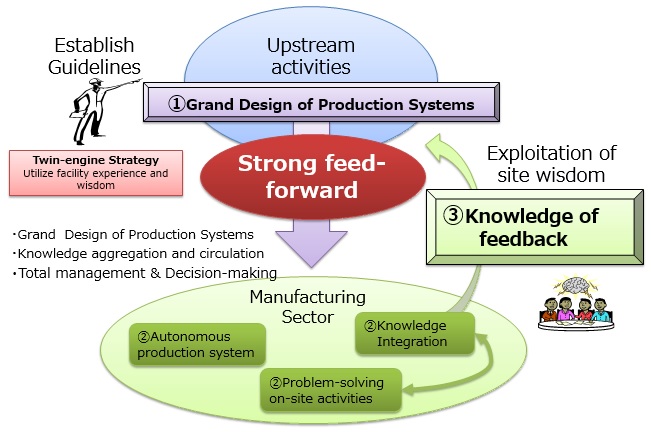
>”Production System” and “Production Model”
Here, a “production system” refers to the mechanism that create goods. A symbolic representation of such a system is henceforth referred to as a “production model”.
A production model is made of the production processes and production resources. Production processes, which comprise a “workflow” whereby goods are created, have an input and an output. The process working with said information is defined as a network structure representing the activity of manufacturing goods.
In order to implement a production process, some static resources are required. These static resources can include but are not limited to a floor plan representing each process, its machinery, and location in a facility, the layout of the plant, an established roster of workers and/or means of internal transportation, tooling, as well as an assortment of dynamic resources.
After all this is established, the production model consisting of these processes and resources is defined as a mechanism that can create this product. In such a production model, products are produced in an ideal fashion from the given resources.
By defining such a production model based on these “systems” ahead of time, it is possible to operate real factories following these guidelines with a knowledge of how the systems will react. This has the benefit of avoiding a large swath of problems caused by haphazardly changing a production model. As these systems are “perfect”, issues such as quality and machine degradation may not be discovered through such a model, but important logistics data pertaining to the manufacturing process such as productivity, utilization, capacity, in addition to more business-oriented data such as product lead time and volume can be measured. Knowledge integration using such data is indispensable in avoiding some of the pitfalls of high-variety, low-volume manufacturing.
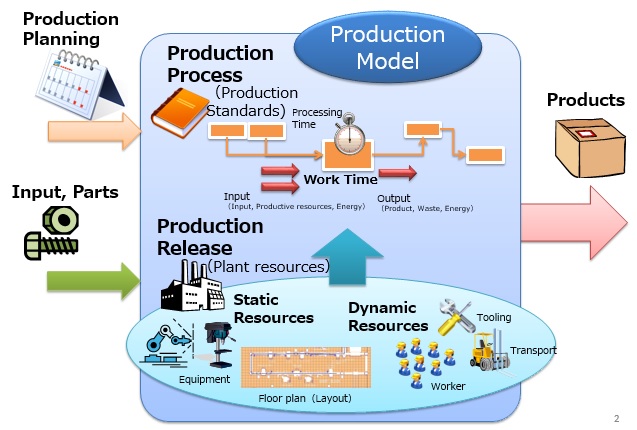
Amalgamation of the Engineering and Supply Chains by "SIM"
In this fashion, the purpose of perceiving the manufacturing system from the perspective of the production system is to clarify the root mechanisms of manufacturing. This will enable accurate control of the direction in which production is headed. By working with production activities in a model-based fashion, it is possible to operate even when the produced goods and marketplace are rapidly fluctuating. Furthermore, it worth considering the situation wherein a variety of organizations constitute the foundations of manufacturing.
In order to implement “production models”, production simulation technology is indispensable. Instructions for production simulations and assignment of resources is performed by a computer, whose behavior is implemented by such technology. Upon logical evaluation of the early production models, the computer will learn how to verify the effectiveness and performance of the production system independently.
By leveraging the production simulation technology, realization of an ideal production model should be possible. This concept is termed SIM (Simulation Integrated Manufacturing). By exploiting the calculation potential afforded by simulations to its fullest, the technology enables users to quantitatively predict the result of employing a specific model. From here on, analysis of quantities associated with trading volume such as productivity, usage ratios, effects of Work-In-Progress, space between logistics and operations personnel, and inter-process procedures will continue.
By employing the concept of SIM, both the engineering chain and the supply chain can be worked with as one unit. By using SIM, as a consequence of dealing with the engineering chain and supply chain as a single unit, rather than considering an “ideal production model”, a “future production model” is considered.
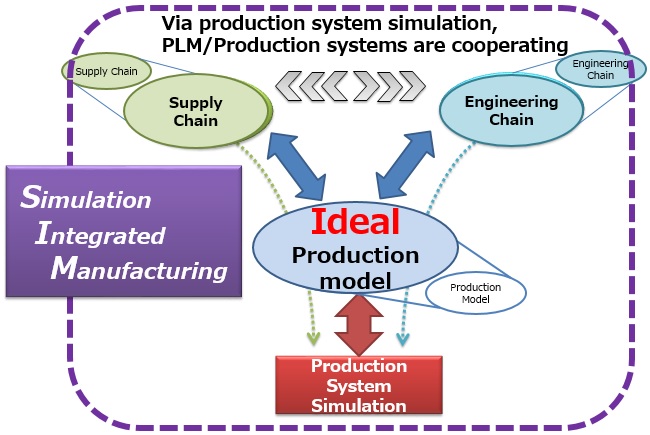
Consider the engineering chain and the supply chain. As there is no IT infrastructure backing the engineering chain, examination leads to the conclusion that the supply chain and engineering chain are unable to cooperate, and so they are currently dealt with on an individual basis.
At SOP (Start of Production), the assorted components of the production system come together. It is at the moment just preceding the end of the SOP phase wherein a problem in the integration between the systems can be observed, and “improvement” performed. Although “improvement” is reputed to be an excellent technique, the circumstances under which it can be performed are far too late and result in considerable effort to perform the rework.
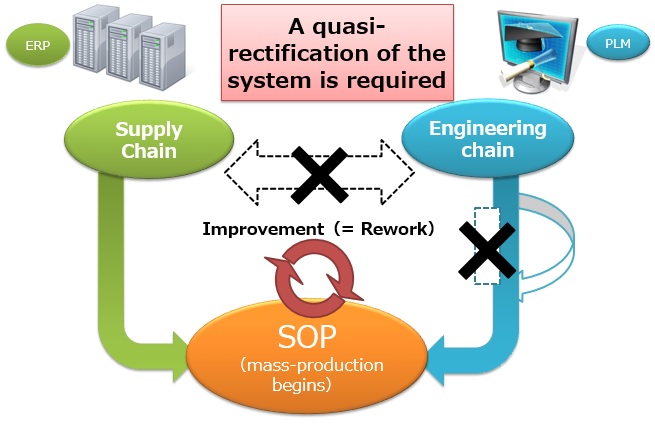
In order to avoid this problem as much as possible, thorough elaboration in the field of upstream planning is necessary. By integrating the engineering and supply chain by using SIM, the rectification of the production process will be accelerated.
What is described here is “Rectification of Production Preparation”. As shown in the figure below, by linking the flow of the engineering chain and the supply chain, operations modifying any of them will be reflected in the other. Traditionally, they consisted of planned activities which were performed individually. By sharing information between the two processes, modifications can be made concurrently.
In order to achieve this, the idea of a “production model” is introduced. By placing the production model at the heart of a facility, production setup activities and information can be controlled simultaneously. As such a change in paradigm can have a slew of effects, a few of them will be described below.
Firstly, the improvement process can be “front-loaded”. In the traditional approach, any problems encountered during mass-production preparations were required to be addressed by “improvement”, just prior to execution. Utilization of the “production model” paradigm allows the tasks associated with improvement rework can be front-loaded. By addressing these problems earlier than would be possible with improvement, they are dealt with well ahead of schedule.
Secondly, production resource utilization is optimized as a consequence of concurrently planning the steps taken in the engineering chain and supply chain. As the production model exists in at the center of the procedure, a wealth of information concerning both viewpoints allows the requirements to be examined at every stage and addressed accordingly. Such information allows for the maximization of productivity and operating ratios while simultaneously minimizing expenditure of production resources and inventory. This affords users of such a method the ability to dedicate more energy towards strategic planning.
Thirdly, it is possible to apply feed-forward to the production sector. In Japan, feedback is often employed, but it is common practice to not employ feed-forward. However, feed-forward is becoming increasingly effective in the area of global business development. It is important to act upon manufacturing concepts, ideas, and guidelines with conviction.
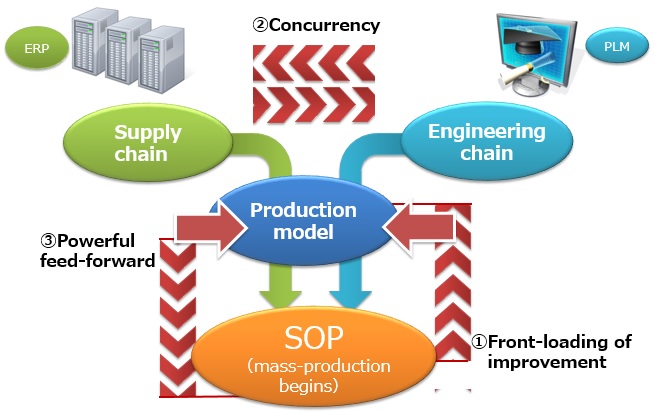
Supporting Upstream Planning Using "MN Simulation"
By employing the concept of SIM, we shall consider how to perform upstream planning. Regarding the production model, utilization of simulation data will help show that the problem exists, but it cannot be solved in such a manner.
In order to solve such a problem, knowledge of the engineering and supply chains are required to help understand the inner workings of a production system. By integrating these fields of knowledge with the simulation data, the problem becomes solvable.
This approach requires unification of two separate models which are contained in a representation of a production facility. The physical components of the facility such as station layout, production process models, and production planning models must be combined with logistical components of the facility such as worker assignments and control of tooling resources. The combination of the production process model and the activity model into “One Model” is crucial to solving this problem.
GD.findi is a simulation engine developed by Lexer Research focused on integrating these two aspects of a production facility into a single model so as to solve the problem at hand.
However, the successful realization of the “one model” does not mean that the problem is solved. Because multiple model systems are condensed into a single representation, a variety of relationships between the components are established in this creation process. As it were, a higher-dimensional solution space arises as a result of this amalgamation. The true solution to the problem arises as a combination of an instance of the problem in each of these higher dimensions. Among all the permutations of selecting one representation in each of these solution spaces exists the true solution of the problem with which we are tasked.
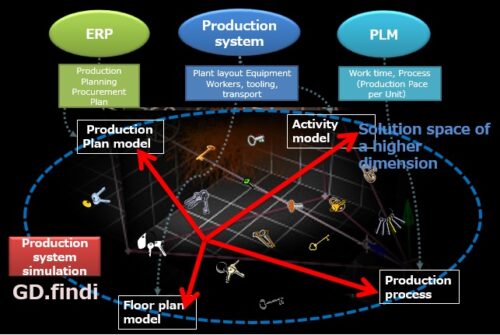
In the search for the solutions, it is important to remain conscious of the current way of thinking. In the higher-dimensional solution space, a practical evaluation of the combinations of each element in the model is required. This combination of each of the elements is termed an “M x N simulation”. Because GD.findi’s representation of a model encompasses all of the models, evaluation of the relationships between the comprising models in the “one model” is simplified.
However, due to the nature of such a solution space careful preparation of each potential solution in the both of the model space is of utmost importance. If this seemingly trivial step is neglected, excessive creation of potential solutions, pareto-optimal or otherwise will occur and result in a combinatorial explosion of evaluations.
To counteract this problem, existing resources such as PLM and the production system theta base are employed to generate the elements which will be permuted within the M x N simulation. The entire simulation procedure is asynchronous, whereby potential solutions are generated at the same time that permutation of said solutions is occurring. At this stage, some sort of generation logic for the potential solutions in each of these spaces are required. As the circumstances governing each facility are inherently different and have until now required individual attention by consultants, extensive development has been put into solving of this problem.
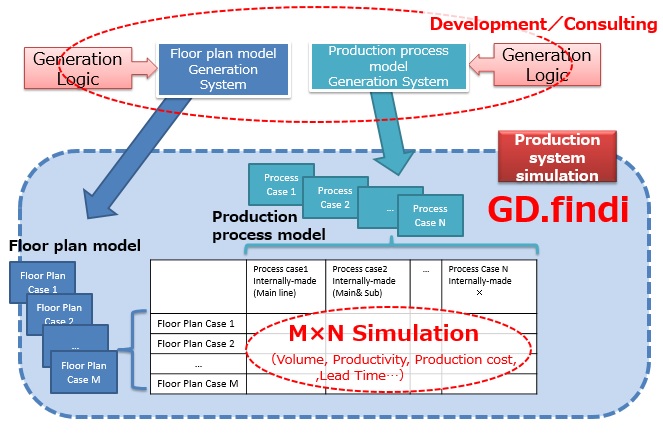
Aggregation of "manufacturing knowledge"
Up until now, there has been discussion on the introduction of the concept of SIM, production models, and the “brush up” procedure. However, as it is nearly impossible to create an outstanding production system through a mathematical approach, such techniques become indispensable.
What is important here is viewing the problem in its actuality as well through the lens of history – to draw upon the wealth of knowledge afforded by a professional and apply it to the problem at hand. Unfortunately, the scale of this problem is such that the mere act of knowledge integration is not nearly enough to generate the desired outcome.
As mentioned previously, the shortage of “professionals” is rapidly approaching a crisis-like state. Combined with the fact that it is already hard to leverage the abilities of the few remaining professionals and the tendency for these professionals to become overly specialized in a given field, the prospects for the future appear grim.
The current state of affairs has resulted in the inability of these uncommon professionals to interact with qualitative and quantitative components of the problem becoming a commonplace occurrence. It has become apparent that from here on, a strategic approach to manufacturing whereby these uncommon professionals can conglomerate their knowledge rather than sequentially offering insight is of utmost value.
As such, examining the manufacturing industry as a whole and utilizing the SIM process to aggregate knowledge of these scattered professionals so as to create an ideal production facility will require development of a new planning process. Within this line of thinking, it is important to note that a database of documented individual findings does not constitute the aggregation of knowledge. Without prior knowledge of the conditions of execution and an understanding of the phenomena resulting in said data, the information stored within such a database cannot be leveraged. A brief analysis of this state of affairs suggests that by working with SIM, a design strategy addressing these concerns can be realized.
In light of these circumstances, because it is possible to combine the wisdom of these scattered professionals by use of SIM it is apparent that the development of a platform whereby this wisdom can be accumulated is necessary. The collective intelligence of these various professionals becomes collective wisdom whereby a wealth of problems can be solved, and so several models of applying this collective are discussed below.
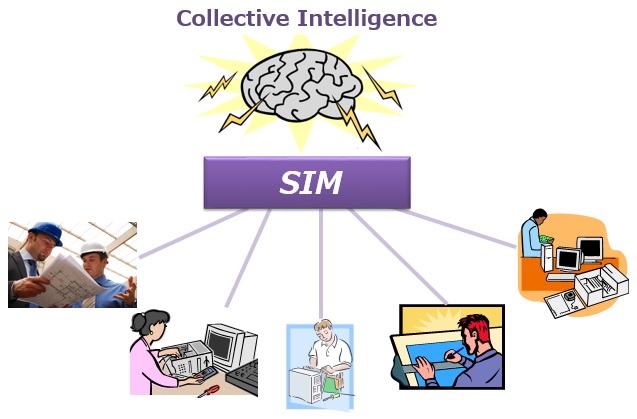
Applications to Business Processes
In this section, the application of SIM to a business model will be examined. A “production system” grounded in production model fundamentals will be considered, and the role of SIM will be to verify its efficacy.
Firstly, it is important to understand business processes of manufacturing which must be undertaken prior to the start of production. These process and tasks consist of budgeting, production strategy design, product design, sourcing parts and raw materials, evaluation, selection and deployment of production line assemblies, and manufacturing schedule development. Only after all of these business-specific processes have been completed can the actual manufacturing process begin.
Upon consideration of this situation, in the event that there is a specialist in each of these fields present for a specific project, then it stands to reason that these specialists will most likely be absent from all of the other projects occurring during the same timeframe. Additionally, it is important to note that many of the business processes associated with manufacturing are heavily dependent on each other, yet are handled by separate business entities. For instance, a change late in the product structure late in these preparatory stages would have a significant impact on product design, tooling, and ultimately productivity of the production process. A significant portion of work would have to be redone to suit the new product structure, which could be devastating if the work was contracted out to a separate business. Up until now, process design has progressed solely by means of the expertise of individuals, and communication of knowledge between these individuals remained largely unexplored.
As such, it should be possible to apply some of the concepts employed when dealing with production systems to other domains which share some background similarities. In doing so, it is possible to construct a manufacturing strategy which produces high quality goods whilst maintaining maximum efficiency.
In the event that SIM could aggregate all of the professional know-how in each of the businesses associated with establishing a production process, it would provide amazing benefits. For example, communication of a raw materials supplier with a product design firm could provide insights which might reduce cost or contribute towards a higher-quality product. The efficacy of such knowledge could be bolstered further by use of production simulations, to create what is ultimately the ideal workflow for production facility design.
Any savings in costs, could, by further application of the collective wisdom, be re-invested into the process to further improve productivity and quality of the facility. As such, using the collective wisdom as a guiding principle in development of production systems can have dramatic results.
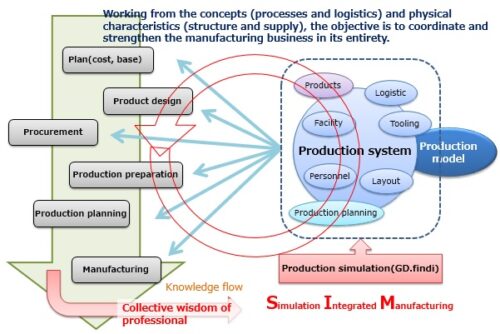
Applications to EBOM and MBOM
In this section, we shall consider the relationship between MBOM and EBOM and its impact on business procedures. Management systems such as PDM and PLM have already been implemented to manage MBOM and EBOM, however these types of systems deal purely with data.
Until now, there was no concrete method for working with EBOM and MBOM. The procedure relied on professionals drawing upon past experience to produce any results of value. With respect to process design and production line setup, only the data produced by a solution that comes into existence is stored.
It stands to reason that there is a cause for certain iterations of MBOM to not reach the production stage. In the event that the MBOM is incomplete, then the associated plan will also be complete. In reality, if an MBOM is not sufficient for a certain project then it will be reworked until it eventually becomes sufficient and reaches the production stage.
Is this practice acceptable in this day and age?
To answer this question, we consider the application of SIM in constructing an MBOM.
EBOM can be obtained from the product configuration information, which is an output of the product design stage. MBOM is necessary in conventional manufacturing processes is typically defined by experts making use of their past experience. Alternatively, the MBOM can be derived from studying the flow of production.
By taking advantage of SIM, it is possible to prepare the MBOM at an earlier stage in the planning phase. Because SIM employs a virtual production line, it is possible to simulate the effects of such an MBOM without a significant investment in physical resources. At this stage, a hypothesized process and layout configuration can be combined with an equipment plan and quantitatively verify both productivity and feasibility. In addition, as it is possible to leverage the knowledge of other professionals, being able to further refine a chosen design is highly likely.
In the context of MBOM development, SIM does not function is a mechanism which automatically generates a solution. Rather, it can be likened to what an IDE does for a software developer – provide an environment which facilitates creation of an ideal solution.
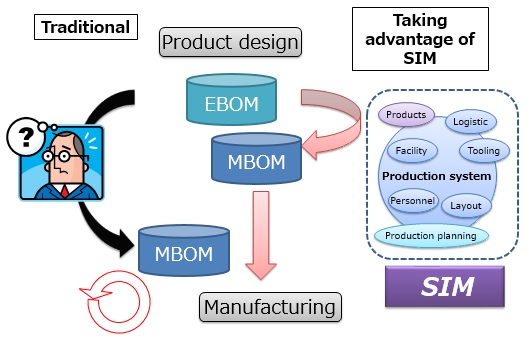
Preparations for Production on a Global Scale
Consider the interaction between business processes within the domain of global production. Global production requires a plethora of planning and resources prior to execution, such as base strategy, facility and equipment procurement, cost and logistics planning, and assorted human resources. Key issues concerning the global manufacturing process which cannot be ignored include early stage production metrics, productivity, and quality.
The business processes associated with starting overseas production tend to utilize resources available in the desired production location to their fullest. However, because there tends to be a dearth of experience and know-how available in these locations problems tend to arise. It is noted that frequently, when management from headquarters come to examine planned facilities that the problems are noticed and a rework is subsequently scheduled.
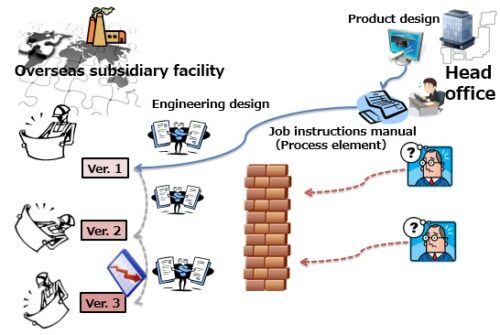
When SIM is utilized as a cloud-based solution, in addition to being able to perform a number of autonomous procedures required for establish production, staff at a company’s headquarters as well as staff abroad can share any information. In doing so, the startup process is facilitated and many of the problems can be addressed much earlier than they would have been otherwise.
Local office staff can verify the feasibility and productivity of a solution using the SIM, and draw upon insight to further refine it. Additionally, staff at company headquarters can share the planning results as well as offering guidance prior to signing off on a project.
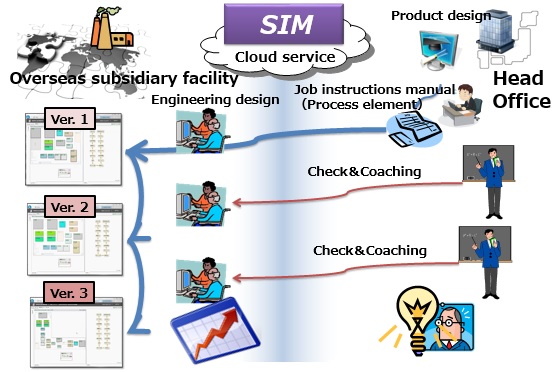
The use of SIM in such overseas production startup procedures may give rise to a spike in such activities occurring, as the procedures are often heavily interconnected. Additionally, as the dispersion of knowledge between project stakeholders is facilitated, the use of SIM in this manner can help guide the strategy of the manufacturing company in global-scale production.
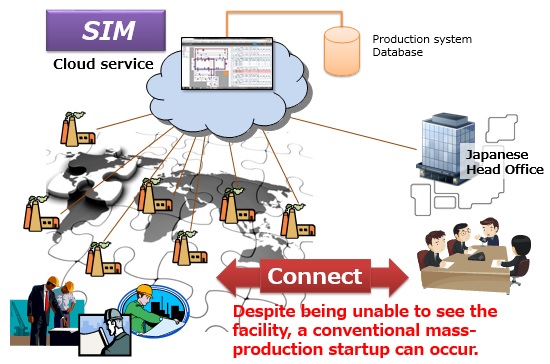
SIM and the Global Factory Repository
In the previous sections, SIM was discussed as a tool to assist in global production preparations. However, it can also be utilized as means of strategically reviewing a global production base.
SIM functions by taking advantage of ERP and databases of PLM information. The express purpose of doing so is such that SIM can draw upon the design and performance data associated with existing manufacturing facility realizations. This “Global Factory Repository” enables access to big data sourced from the manufacturing industry.
By employing the concept of an “Imported Factory” to serve as a seed in determining solution candidates using SIM, it incorporates elements of an already valid solution and focuses on improving it with respect to the problem at hand. The outcome of such a process is termed the “Exported Factory”.
As a result, the data pertaining to an exported factory will meet certain effectiveness and feasibility requirements and has the advantage of reusing similar technology used in the imported factory configuration.
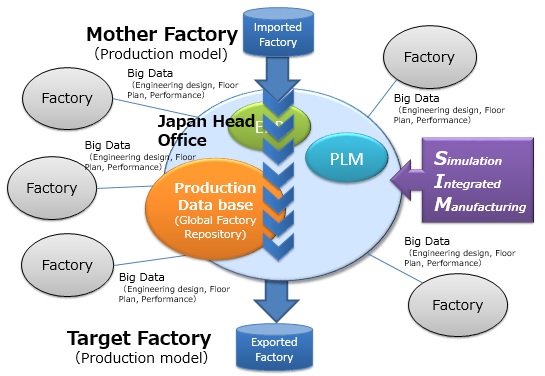
Production System-Sourced "Manufacturing Production Strategy"
SIM has a lot of potential to improve the fields of production preparation and global production by providing information such as the strategic review of existing facilities. However, there are no other approaches known to be able to leverage deployments of production system designs to improve manufacturing production strategies.
Traditional production strategy is a misnomer, as it does not consist of a strategy at all. Technology strategy, product strategy, and all other strategies employed within production were developed within accordance with sales strategy. The historically strong manufacturing sector was supported by heavy reliance upon subcontracting facilities. Was this really a good idea? No, that is most certainly not the case.
As it stands, the manufacturing sector does not presently lie at the bottom of the “smile curve” – that is to say, there is still potential for a great deal of improvement. For the well-being of the manufacturing sector, it is crucial that this fact is capitalized upon and progress made towards realizing said potential. In order to do so, SIM should be applied to as many businesses in the production sector as possible so as to make apparent what the best practices are and how the objective “best” facilities utilize them. In doing so, the untapped potential of the manufacturing sector is exposed and can be seized.
Up until now, the paradigm of the manufacturing industry has been to design a factory that meets a specific production target. However, given a single plant, would it not make more sense to take advantage of it to its fullest, thereby bringing about a surplus in produced materials? This net positive in produced goods, be it however small for each factory, can surely be aggregated over time and over many facilities to affect a firm’s business strategies.
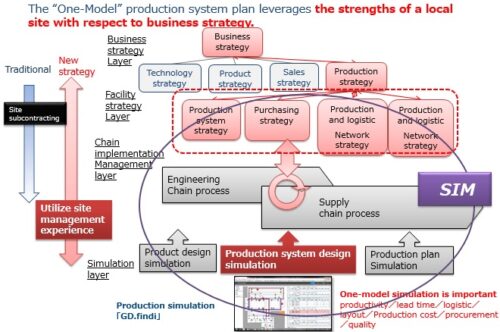
In the "Industry 4.0" era, professional wisdom is mandatory.
As the subject has changed considerably, let us consider the ideas of Industry 4.0 and the IoT. The future of the manufacturing industry is closely tied to this technology and the idea of a “smart factory”, and it will be impossible to continue to operate while ignoring these concepts. However, the mere introduction of such “smart factories” does not address any of the issues that have been discussed here.
The diagram below illustrates the concept of Industry 4.0, and how the equipment and personnel in a plant can exchange information by connecting to a local network. This establishes a mechanism whereby dynamic control is established, creating intelligent functionality on the production site. Ultimately, the goal of such an idea is to help meet the demands imposed by low-volume, high-variety manufacturing. In addition, the ability for individual “smart machines” to autonomously integrate themselves into the production process by PnP (plug and play) adds to the modularity and flexibility of the production system.
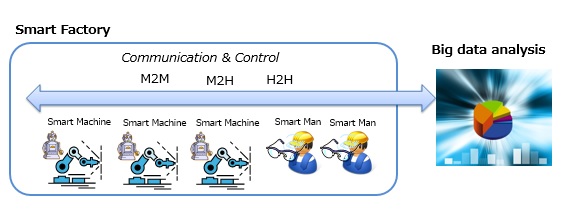
It is beyond a shade of doubt that the existence of such a production facility is innovative. However, the mere existence of the smart factories and prevalence of big data analysis is not satisfactory.
For instance, consider applying these concepts and creating a “smart” version of a traffic network. As things stand in the present, human beings are responsible for driving a car. However, in a “smart” transportation network, instead of humans controlling the cars, some type of robot or microcontroller is responsible for controlling the cars. These robots or microcontrollers are connected to the traffic network, and so the principles of IoT apply.
All automatically-controlled cars in the environment can communicate with each other as well as the traffic signals. Information from other vehicles and the traffic signals are used to coordinate the movements of each individual vehicle.
It is quite clear that a problem arises as a consequence of such actions, however. Likening a smart production facility to this smart transportation network, the performance metrics of traffic capacity versus production goals can be observed. However, the typical state for a production facility is “lean”, whereby the production targets are barely met. This state of affairs in the context of the smart transportation network would be limiting the total traffic going through the system at any given moment in time.
If this were to occur, a traffic jam would ensue. Even if both the automatic driving behavior and the ability to communicate with the traffic network were perfect, it would be impossible for the traffic system to go beyond its capacity. The inability of such a network to perform actions such as pulling over and locating a less-crowded detour is a possible shortcoming of such a solution.
There is also the chance that certain corner conditions may be met with such a system. For instance, a very tall or heavy truck may be unable to fit through a certain tunnel or bridge, or perhaps be too wide to enter certain streets.
It is important to note that the delegation traffic control duties onto this smart transportation network may have some benefits, but in doing so many of the untapped benefits resting within such an approach remain inaccessible. By incorporating some affordances into the planning phase of this transportation network, the overall performance of the system can be increased significantly in the event that some otherwise problematic situations are alleviated by virtue of good design. The objective is to take advantage of the flexibility of the smart transportation network.
Of course, this brings to the forefront the non-trivial task of designing a transportation network to begin with. As has been stated before with respect to the domains of manufacturing, leveraging traffic simulations and big data techniques here will help provide insights as to the behavior of such a system, and so is important.
Additionally, it is important to collect information relating to actual implementations of these systems so that principles underlying their behavior can be observed, analyzed, and drawn upon. It is crucial to note that the motivation behind such a strategy is not with the goal of analysis, nor problem-solving. Rather, it is about understanding the purpose for which a system is designed so that an ideal solution may be created.
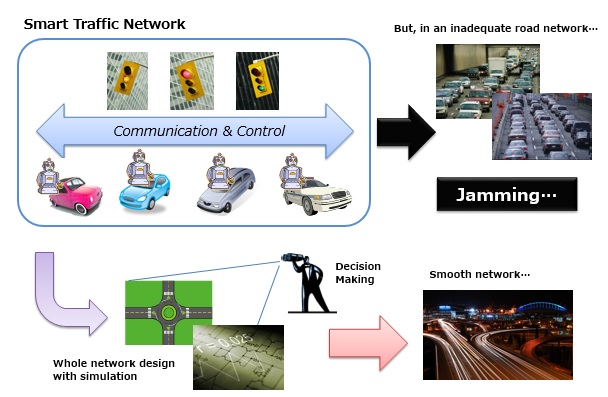
All of the principles touched upon in the smart transportation network example are likewise applicable in the smart factory scenario.
Smart factories clearly have a wealth of potential, however in order to utilize these capabilities to their fullest the design of such facilities must be performed with utmost care.
This entails that considerations should be made regarding process, assembly line, facility layout, and logistics design so that fluctuating production targets can be made. In addition, some considerations should be made with respect to personnel and tooling such that they can adapt to these rapidly changing targets. The result of such flexible behavior is that even if the circumstances of production were to change, the facility would still be able to operate to its fullest.
SIM can play a vital role in the design of such a factory network. The behavioral characteristics of “smart” personnel and machinery in a production system can be implemented virtually to aid in predicting the behavior and condition of plant operations within the smart factory, thereby imparting information enabling a designer to refine or select a solution.
The most precious component of SIM, as touched upon previously, is the addition of the “wisdom of professionals”. By using SIM, it is possible to generate a number of mathematically proven candidates for a specific scenario. However, because mere mathematical figures are limited by the nature of the software and the vastness of the solution space the input of a professional in the field is still essential.
As such, it is still necessary to collect both the data from existing implementations in conjunction with the knowledge of the professionals who have worked on such implementations.
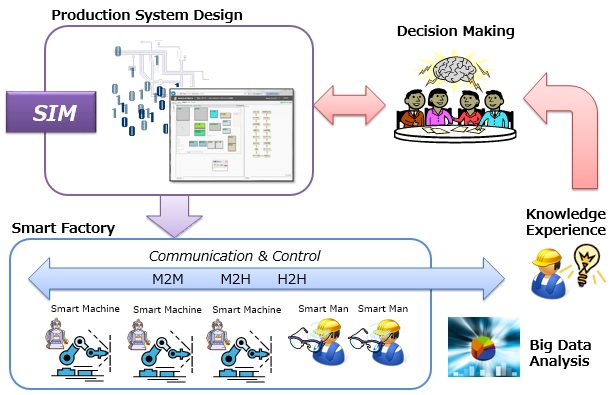
For the purposes of leveraging Industry 4.0, Internet Factory, and the other concepts discussed here an assortment of devices, systems, and tools have been developer and available for use.
As proficiency in designing smart factories and performing big data analysis increases, so will the sophistication of these techniques. It will be necessary to, alongside these techniques, develop a set of techniques whereby the “wisdom of professionals” can be used to aid in production system design.
Upon the base of SIM, we are developing and intend to offer mechanism where by “Collective Intelligence” and “Collective Wisdom” can be aggregated.
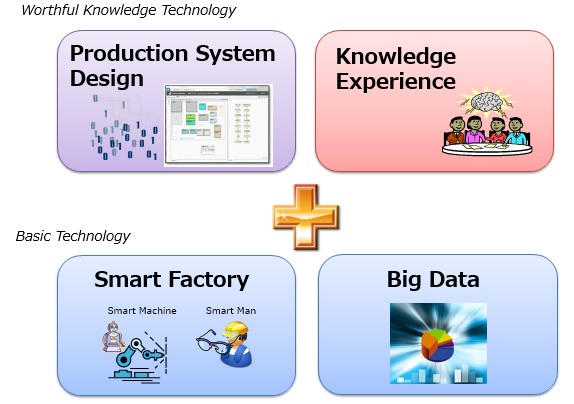
Virtual, powering everything!
It is important to consider precisely what is entailed by “virtual”. In this case, it is not merely a computer-based substitution for reality. Rather, it means “functionally as is desired”.
In this sense of the word, if we consider chess, a similar game with some changes made is virtual. A low-fidelity prototype from a 3D printer is virtual. A production line consisting of cardboard is also virtual. Should the object in question meet its intended requirements for functionality, it is virtual.
The things in question can be real, and working, or they can be imitations. They can be physical or virtual, in the sense that they exist only inside some sort of runtime. All that is required for something to be virtual is the fact that it works as is functionally desired.
And so, by creating a slew of virtual environments, we can come up with a myriad of designs that meet functional requirements and can serve as the starting point for a solution.
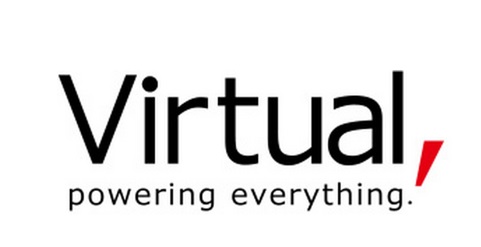
We, in order to further refine production systems in the manufacturing industry, have embraced this virtual technology wholeheartedly.
Our goal is to create a functional environment in which people involved in any part of manufacturing can get involved, obtain knowledge and understanding, and collaborate so as to propel the industry further into the future.
Leveraging the overwhelming power of virtual technology to handle all aspects of manufacturing design, light is shed upon its future role in this industry – powering everything.
For product information about GD.findi MS, a production system simulator that utilizes GD.findi Data Model, please click the following button.